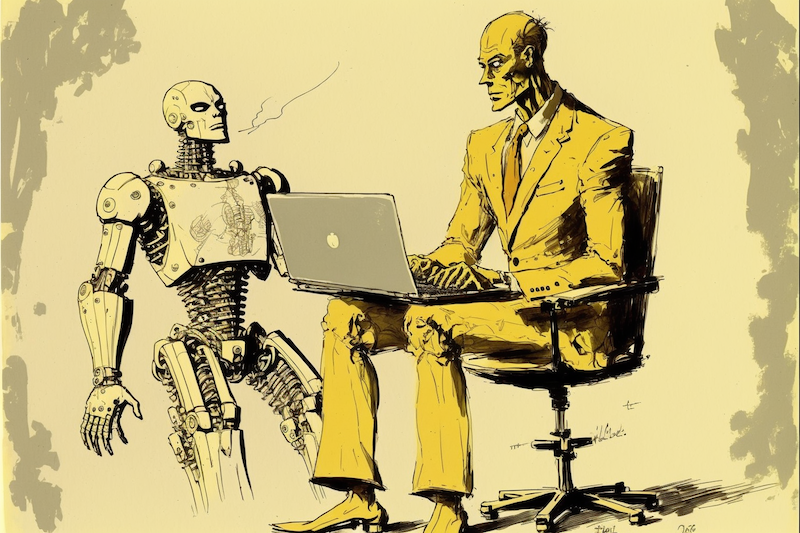
Digital Democratization: Tech Osmosis
Following on from a recent post about digital democratization, let’s explore where Generative AI fits into the picture in order to arrive at a more interesting use case for Large Language Models (LLMs) like GPT-3, ChatGPT and Github Co-pilot.
Citizen Coders and Citizen Technologists (henceforth both called Citizens) are vital for the future of digitally complex enterprises. I have argued that the only way to accelerate digital dividends is to amplify the efforts of workers closer to the customer and/or closer to the in-flight business problem.
Indeed, per Gartner, CEOs increasingly hold the belief that the answer to unlocking greater digital dividends is placing more technology into the hands of business operators. They expect their CIOs to deliver.
The reality of modern software tooling is that it is often easier for a business-domain worker to gain access to automation via new tools (like embedded data science — e.g. in Tableau — or no-code super-apps or reverse-ETL) than it is for a highly technical engineer or data scientist to grasp the in-moment need and prioritization of an in-flight business problem. This is thanks to the ongoing march of automation.
There is a kind of digital osmosis whereby power flows more easily from technology to the business domain than business knowledge can flow to the technical domain, if it isn’t lost in translation.
This is especially true under conditions of uncertainty and complexity that are here to stay in the modern enterprise.
Let’s put it another way: if a business worker has to spend more effort explaining and justifying a biz problem to a data scientist, data engineer or salesforce operator than they could theoretically spin up their own solution using self-serve techniques, then you’re already too slow and likely to suffer the dreaded Red Queen effect that business strategists like to remind us of.
Generative AI and Citizen Productivity
A key automation force will be Generative AI in the form of code generation (e.g. Github Co-pilot) and bot-like interfaces like variants of ChatGPT.
Indeed, I predict that the sweet spot for these technological possibilities will come when Citizens are able to create their own hybrid-chat interfaces — think thousands of helper bots, or chat-powered UIs (with embedded low-code widgets), not one giant “Siri for the enterprise”.
Over time, Generative capabilities will widen to include more tools. For example, in a recent assignment for a major digital experience vendor, I proved that it is possible to generate website pages using the same Transformer technologies that underly LLMs. We know that start-ups like Adept are working on generalized UI-learning machines in the same manner.
There will be several outcomes that accelerate digital dividends:
- Citizens will become 10x more productive at solving in-flight business problems.
- $-for-$, Citizen productivity curves will outstrip the productivity curves of in-house technical specialists.
- Specialists will migrate to core technical innovation tasks, such as customized AI model production which, in turn, will empower Citizens.
- The delivery model of specialists will increasingly be via generalizable Citizen-accessible interfaces.
Accelerated Context Switching
A key part of the puzzle is knowledge management. Over time, the ability for Citizens to become successful will depend upon their ability to understand various moving parts (like datasets) quickly. Generative techniques will somewhat lessen the burden of having to understand details, leaving that to models that sit in constant fine-tuning loops.
Citizens will recognize that their ability to be successful will depend upon context-switching efficiencies — how easy it is to switch from one task to another.
As I argued elsewhere, the great potential for apps like ChatGPT is a “mental un-blocker” apps that can quickly bootstrap a worker into a knowledge space. So, these technologies will help Citizens with task-switching.
Task contexts might include things like a set of parameters or instructions to set up a data flow via reverse-ETL into Salesforce, or the meaning of a Snowflake model or, the perennial problem of where to find data.
Indeed, at a certain point, the emerging data semantic layer (e.g. see Semantic Layer for DBT Cloud) will be totally subsumed into LLM-based apps to make data more amenable to semantic search.
Apps like ChatGPT will not replace data analysts, but will increase the productivity of those attempting analysis who do not spend all their time with the data, like a Citizen Coder.
Eventually, Generative AI tooling will allow business folks to operationalize the generation of insights “at the speed of thought” — an oft-mentioned phrase, but seldom achieved.
In terms of allowing easier access to data in the first place, the challenge for data-tools vendors will be to make their wares “LLM-indexable” — i.e. easily accessible via natural language querying. (This will be part of the emerging Data Fabric architecture, although many vendors are seemingly behind the ball with this realization.)
Gig Citizens
In working with Generative AI tools, Citizens will learn to adopt a new mode of documentation, or knowledge curation. We can’t say what this will look like, as it hasn’t been invented yet — we are still adjusting to the way interfaces like ChatGPT work and very few of us have had the opportunity to fine tune them to include our own knowledge.
I predict that Citizens will learn to write “notes to self” that are actually “notes to self via bot” — i.e. document my work in a way that I can pick up the thread again quickly by asking my bot.
Powerful new techniques in data sharing, as available via tools like Snowflake, and new modes of AI in data permissions and privacy protection will empower Citizens to work from anywhere and become specialists: RevOps Citizens, SalesOps Citizens, Growth-Hacking Citizens etc.
This will slowly pave the way for what some CIOs are already wondering: what are the opportunities for a “Gig-Citizen” economy?
One has to believe that the opportunities are vast and that new tools will emerge just to power that economy. Generative AI will play a central role.
Impacting The Bottom Line
Citizen Coding is not a new idea. It is already well underway thanks to the rise of automation and expressive tools like no- and low-code apps makers.
The so-called Citizen Technologist, or Business Technologist, is just an extension of the Citizen Coder concept: someone outside of IT who knows how to drive technology to get results. A good example would be someone who knows how to set up a workflow using Zapier.
How will this impact the bottom line?
Any Citizen ought to be working towards a set of KPIs that roll-up via a KPI hierarchy to business outcomes directly related to revenue.
An insight is any data-driven realization that is actionable against improving a KPI. So, if workers are empowered to generative insights for themselves and make workflow adjustments for themselves, this ought to have a direct positive effect upon related KPIs with knock-on improvement to the bottom line.
This is just one way in which AI, in this case Generative AI, will help to accelerate dividends from digital technology investment.
Article written with inputs from Irzana Golding about data democratization and insights operationalization via tools like DBT Cloud.