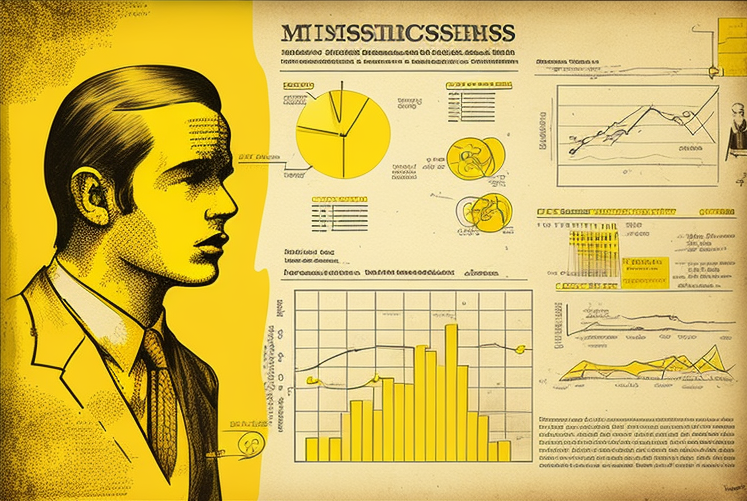
I my last post, I wrote about being “data driven” operationally, and in the post before that I wrote about the mindsets that surround using machine learning models.
Let me try to marry the two ideas.
There is a widely shared mindset that models are somehow free of bias. And I don’t mean statistical bias. I mean prejudicial thinking. We may not recognize this at first because the name “data science” seems to suggest that modeling is a scientific endeavor.
Let’s say it is scientific, then what kind of science?
These methods, as used in enterprises, are often closer to social science.
I say that in the sense that we are often trying to account for behavioral mechanisms (in the enterprise) using statistics.
The hypotheses formed about the enterprise are theories about human activities, not, say, balls rolling down a slope (laws of physics).
As such, business leaders need to be aware that this entire gamut of activities can easily be gamed to make the statistics say whatever we want.
A damning analysis of how this happens is well documented by Gerd Gigerenzer in his paper: “Mindless statistics” — the inspiration for my article’s title.
In it, he gives an account of how a statistics textbook written by a university professor was revised to remove a broader discussion of statistical techniques in order to focus on only one. When asked why, the professor said:
Most researchers […] are not really interested in statistical thinking, but only in how to get their papers published.
We could easily substitute this for: “Most managers are not really interested in statistical thinking, but only how to get their ideas accepted.”
This reality is what data-driven operating models need to address.
Yet, the discussion is often about “Data Governance”, or the like, as if assuring quality and control of data will translate into statistically-valid decisions.
Perhaps in the oft-quoted 5 Vs of Big Data, there needs to be a sixth V: Veridicality, as in coinciding with reality.
There are no easy answers here.
We can wave our hands about solutions, yet the statistical-heuristical dichotomy pervades how we operate our businesses.
I can say two things as food for thought:
Nurturing a Numerical Culture & Systems Thinking
First, as mentioned in the original mindsets-post, all processes are socio-technical in nature. Social pertains to culture. Before we talk about AI-first, we need to talk about building a data-first culture, possible even with the humble spreadsheet or, heavens-forbid, a whiteboard or napkin.
We should all be hands-on with numbers and strive to explain our arguments using numerical reasoning versus our tendency to resort to qualitative narratives. Business leaders should, well, take the lead, and be sure to encourage numerically-reasoned arguments, including causality assumptions.
This is hard to do, but that’s good because it means that it requires System 2 thinking that is supposedly less vulnerable to bias. Its deliberate application to various actives has been fairly well studied (beyond the problematic Thinking Fast & Slow book).
Much has been said about the value of design thinking, with many companies, like IBM, adopting it systematically. But little is said about numerical thinking or its grander counterpart systems-thinking, which is often still treated as a kind of esoteric topic favored by management theorists and Complexity fans.
Just as we should all become design thinkers, we equally need to become systems thinkers. (See this great post by Dave Wells on prognostic analytics and systems thinking.)
Discipline: Start as You Mean to Finish
Still related to culture, leaders have to set the tone about what kinds and levels of discipline they want to operate with. We all know that our behaviors align to incentives. If good data discipline isn’t incentivized, then who’s going to bother?
It is well documented that the manner in which a start-up sets out in terms of adopting good practices is how it ends up. Indeed, they are related because good practices are what makes a business repeatable and scalable. We have known this since Six Sigma, which saved many US companies from the onslaught of process-obsessed Japanese industries.
Yet, just as we learned that you cannot inspect robustness into a product or process (hence Design for Manufacturability), we have seemingly not learned that you cannot inspect robustness into your data processes or culture.
This is still the dominant mindset of so many enterprises and is a reflection of the same lack of strategic mindset (i.e. dominance of short-term thinking — action first, data second) that dogged US industry prior to Six Sigma.
Cultivating a mindset of doing things “the right way” is essential. In some areas, like software engineering, the principles of “the right way” are now well established that they are infused into the wider culture of software participants almost as a code of conduct.
These methods are beginning to percolate into data via the DataOps gamut of ideas, but still slow to penetrate wider organizational behaviors.
I would argue that Design for Measurement ought to become part of Design Thinking. Indeed, like all new things, it is actually an old idea, but still broadly missing from our codes of conduct.